How the United States invests in supercomputing
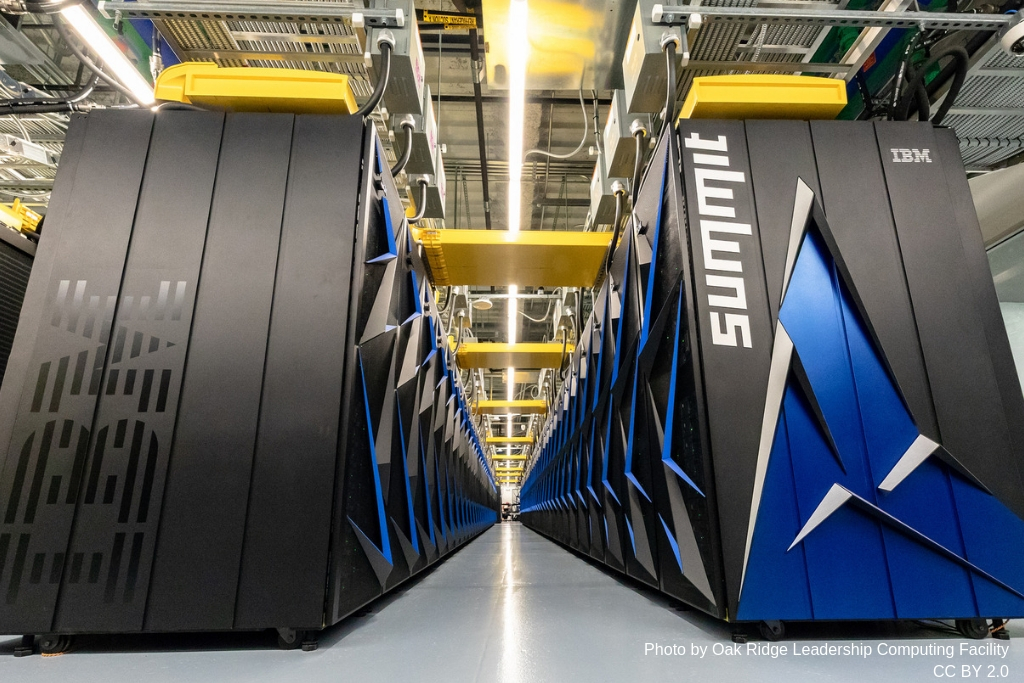
At the end of October, the US Department of Energy unveiled Sierra – the second leadership-class supercomputer delivered as a result of CORAL collaboration between Lawrence Livermore, Oak Ridge and Argonne national laboratories. Earlier this year, Summit was launched. Both machines are among the most powerful supercomputers on earth – Summit is #1 and Sierra #3 #2 on Top500 list. We had the opportunity to discuss the details of the CORAL project with Dr. Dimitri Kusnezov from the US Department of Energy.
Disclaimer: The views expressed in the responses are personal and do not necessarily represent the view of the U.S. Department of Energy or the United States.
Maciej Chojnowski: At the moment, the CORAL systems Summit and Sierra are the #1 and #3 #2 most powerful supercomputers on the planet. In an interview during the SCFE2018 conference in Warsaw, you said that what counts most in HPC is not computers themselves but the purpose we try to achieve using them. What is the purpose of these systems? To what sort of work will it be harnessed?
Dimitri Kusnezov: You are asking the right questions. We use public money to support the development and delivery of these remarkable supercomputers. But they are just tools – so we need to be sure that it is the right tool for the problems people care most about, and that the return on the investment (ROI) far exceeds the cost of these systems – increasingly in the hundreds of millions of dollars. The ranking of the systems should not be interpreted as a measure of the success or overall utility of such a tool. It can be a distraction that drives systems to perform against metrics that do not accurately measure the needed workflow you may otherwise optimize your computer design to.
We do look hard at who will use these systems – years in advance, whether the cloud is a more effective option, whether a collection for small systems is more cost effective, and so forth. It is all based on the classes or problems you believe are worth that scale of investment. These large systems take years to plan, co-design and deliver, and we develop our computer codes during this period so we can test performance on early prototypes prior to full delivery. So to your question, what is the ROI and what will these systems do? Of our two big systems, one is for a well-defined set of nuclear security questions and the other one is for the open scientific and technology community. Interestingly, fundamental and applied science issues are common to both systems, they just answer different types of questions.
I think of the ROI through several measures. For Sierra, we have classes of problems that impact billion dollar class decisions. So it is not hard to make that calculation. For decisions based on simulation, the confidence in your predictions is very important. We call this ‘uncertainty quantification’ or UQ and it can be computationally demanding – more than we can accommodate even at this scale. I described some of this in my keynote last winter at SCFE2018 (which I wrote up in https://arxiv.org/pdf/1804.11002.pdf). For applied science and technology, the ROI is on the order of 500 to 1. That is for every dollar invested, you have a return of somewhere around 500 dollars in productivity or market value or similar. For pure science, you can measure the impact of the work produced and assess whether the results have had fundamental changes in the understanding of key problems: are they the top discovery, in the top 5 or top 10 or less impactful, for example. An objective look can help gauge your effectiveness in using these systems. But this type of return depends on planning the use cases in advance in order to maximize the impact of the supercomputer during its 4 to 5 year typical lifetime in service.
Dimitri Kusnezov, Supercomputing Frontiers Europe 2018 in Warsaw, Poland
The CORAL system designs in Sierra and Summit create “a new breed of computer”, according to NVIDIA CEO Jensen Huang this summer. It is expected to let scientists harness AI hand in hand with simulation. What are the key technological components that make it possible and why is it important?
We are in a remarkable period of technology change today. Artificial intelligence is of increasing relevance to all our activities, across the spectrum from sensor data and detection, through learning methods such as machine learning (ML), to decisions based on searching, planning and proving, to actions such as autonomy or human/AI interfaces. When we started down the exascale path some years back, it was not with AI in mind. But as technologies have developed, we have found that these hybrid architectures are well suited to helping us better understand the ML piece of AI in more detail. For these particular systems, we did push in a number of directions, from the design of the motherboards and water cooled compute nodes, to pushing limits for the GPU resilience, scheduling, diagnostics, burst buffers, switch based collectives, GPFS performance & scalability and so forth. But these are productivity enablers for the larger purpose of taking initial small steps in starting to drive AI into model based prediction. We will begin to study how to augment computer simulation with learning based methods, recognizing that we have a user base invested in traditional computer simulation. So a gentle turn is needed in our large systems. As tools, these systems can help us understand ML in different ways, but we know they are not specifically optimized for that. Today, there is a remarkable global industry developing in novel AI based hardware, designed and fabbed for AI which can offer remarkable speed-ups. We are certainly pursuing that as well.
IBM’s architecture for the CORAL systems integrate the data analysis capabilities of IBM POWER9 CPUs with the deep learning capabilities of GPUs. What are the expected results of this architecture?
Aside from the power efficiency, network advantage of the fat nodes and the complex memory hierarchies, what really caught my eye some years back was the coherent memory space on the nodes. That and the number of PCIe slots. It opened the door for exploration of how neuromorphic or machine learning technologies could coexist with our more traditional approaches to computer simulation. I do believe that the future of predictive simulation will require a bold step into data centered AI approaches and these architectures are suited for taking some first steps in understanding how you integrate machine learning methods with the more traditional approaches to model-based prediction. That is what I really find exciting.
Why is this important? Our department should better be called the Department of Hard Problems, or the Department of Modelling. We drive computing and simulation not as a means to an end, but as a tool to help us answer questions that are consequential and important to get right. From nuclear security to the energy sector and cyber, there are decisions we have to make with limited funds to ensure against situations we hope never happen. Simulation provides us with a means to understand problems we face and provide options. But simulations without rigorous bounds on their validity are not predictions nor actionable. For that reason we have been pushing validation, verification, uncertainty quantification (UQ) and the many methods needed to help bracket our confidence in any prediction. These architectures move us closer to those which will ultimately be able to help us with this – more intelligent, better able to deal with the deluge of experimental and numerical data, and more cognitive. These architectures, while ‘smarter’ will help us start this transition to tackling the UQ problem which I believe to be NP-Hard, in the sense of complexity theory, and consequently problematic on any von Neumann architecture anyway.
In April this year, it was announced that DOE intends to spend $ 1.8 billion on building 3 exascale supercomputers. It will be CORAL-2 program. Both CORAL and CORAL-2 programs mandate architecturally diverse machines: CORAL systems are the results of the collaboration of IBM, Nvidia and Mellanox, while A21 will be produced by Intel and Cray. Why this diversity?
The diversity of the industry and the competition that emerges when we challenge the technology sector with new designs helps drive innovation and is an important part of the technology development cycle. We look for approaches where industry can collaborate on solutions together, leveraging their strengths and product development paths, to allow for novel architectures and hardware/software approaches to otherwise hard problems through such cost and risk sharing. We also do quite a bit of non-recoverable engineering (NRE) work with the industry to help develop technologies that would not otherwise be available for us, and which might be aligned with their technology roadmaps. The larger the pool of companies out there, the richer the set of ideas that emerge.
Thank you.
Dr Dimitri Kusnezov was the keynote speaker at Supercomputing Frontiers Europe 2018 in Warsaw, Poland. You can watch his talk and interview with him in the MEDIA section on the SCFE website.
Registration for SCFE2019 is already open.